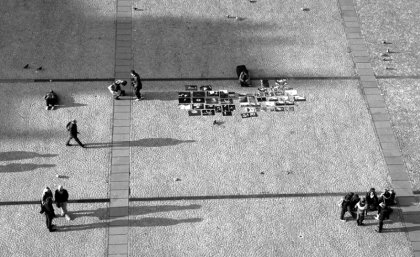
Radim Hladik : « Topic modeling for scientific texts », intervention organisée dans le cadre du chantier transversal Politiques des savoirs
14 décembre 2021 : 14h30 - 16h00
à l’ENS de Lyon, site Descartes, salle D4-145, sur inscription
Présentation
Radim Hladik, chercheur au Centre for Science, Technology, and Society Studies de Prague (Institut de philosophie, Académie tchèque des sciences) interviendra à l’ENS de Lyon le 14 décembre 2021. Actuellement chercheur invité à l’EHESS avec le soutien du Centre français de recherche en sciences sociales de Prague, il présentera une recherche utilisant des outils de topic modelling pour analyser des textes scientifiques (résumé ci-dessous).
Organisée dans le cadre du chantier transversal « Politiques des savoirs » de l’UMR CNRS Triangle et du Laboratoire de l’éducation, cette présentation en anglais pourra intéresser non seulement des personnes travaillant sur la science, mais aussi celles et ceux qui s’intéressent à l’analyse quantitative de textes.
>> Pour vous inscrire, merci de contacter Julien Barrier et Emmanuelle Picard.
Résumé de l’intervention :
This talk will demonstrate the application of topic modeling to a corpus of scientific texts - the publications’ abstracts. From the information retrieval perspective, texts are mixtures of topics, and topics are mixtures of words. Topic models, then, describe the entire corpus by a limited number of ordered word sets, often with an evident semantic interpretation. Topic modeling has been widely adopted by digital humanities, social sciences, and in retrospective review studies of scientific disciplines.
Most applications of topic modeling, however, remain descriptive. We can get more value from the topic models if we promote topics to the empirically-derived units of analysis. A recent network-based topic modeling algorithm (TopSBM) overcomes some limitations of the previous approaches that required researchers to determine a priori the number of topics in the model. We applied the algorithm to more than 80000 scientific abstracts (of books, book chapters, journal and conference papers) retrieved from the database of Czech scientific publications and obtained a solution with four levels of hierarchy, ranging from 52 to 1993 topics.
As will be shown, the resulting topics efficiently reconstitute the space of scientific disciplines. The topics can also be used to determine thematic portfolios of individuals, teams, or institutions and to measure their levels of topic similarity or topic concentration. We can determine the relative femininity or masculinity of scientific topics from the gender composition of authors who publish on a given topic. Similarly, we can estimate the funding levels for each topic in the model from the funding acknowledgements in the publications.
Finally, we can calculate correlations between various topic-level measures. Topic models can be especially useful to discover meaningful relationships from the text data in the absence of additional information, such as when only abstracts and no references are available (typically in students’ theses data). Even for richer datasets, however, it may be beneficial to compare how the topic structure that emerges from the content of documents maps onto document metadata. We are also exploring the possibility of applying topic modeling in a multilingual setting to compare the topic portofolio of projects supported by the Czech Science Foundation and the French ANR, two science funding agencies that do not share the same disciplinary classification.